by Richard A. Davis
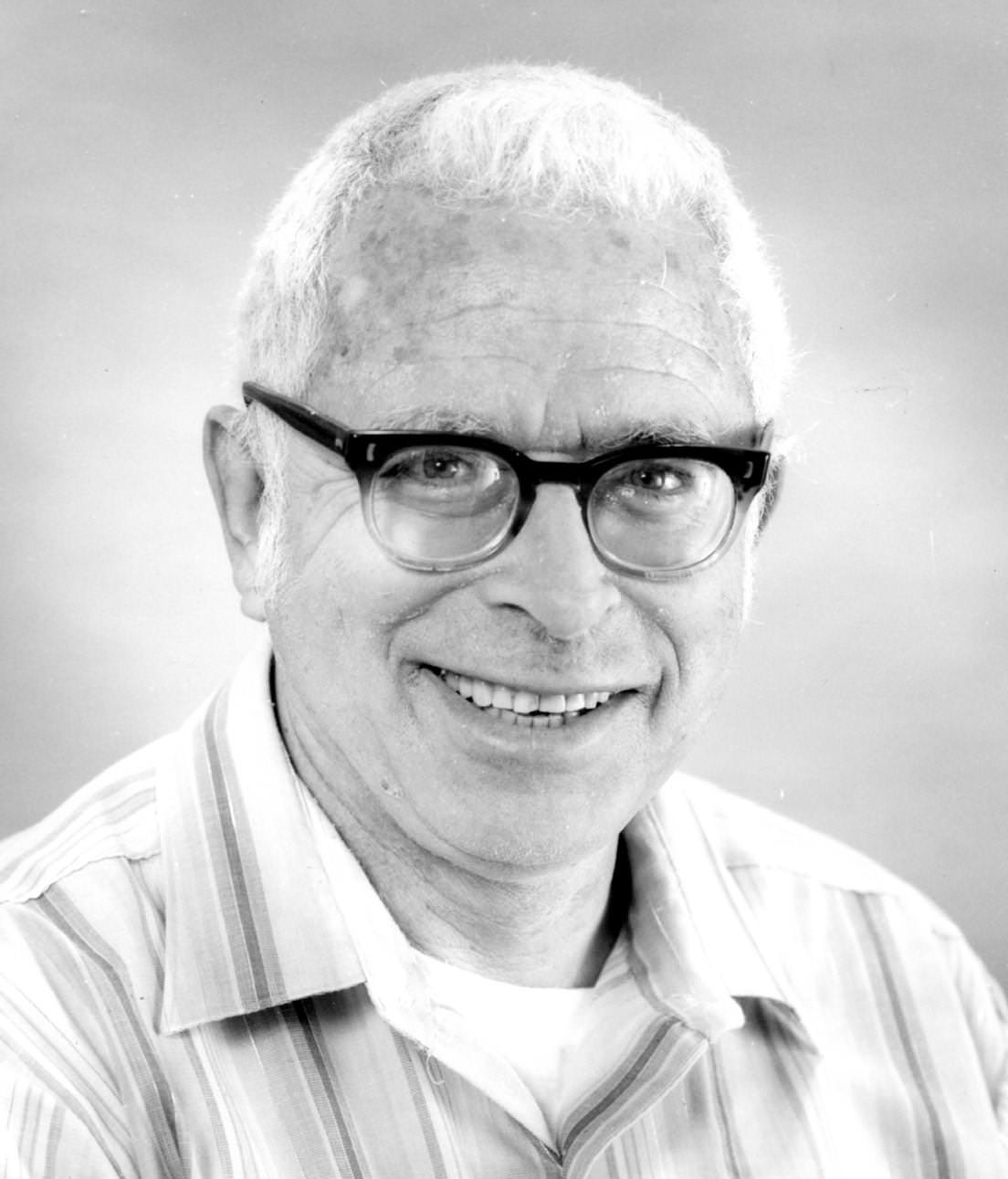
Rosenblatt was born in New York City on September 7, 1926, the younger of two sons of Hyman and Ester (Goldberg) Rosenblatt, immigrants from Ukraine and Poland, respectively. Murray’s older brother, David, and his spouse, Joan, were also mathematicians/statisticians of some note. Both had distinguished careers working in the federal government, with Joan spending her entire professional career at the National Institute of Standards and Technology (formally the National Bureau of Standards). Murray graduated from high school at the age of 16 and then proceeded to study mathematics at City College of New York (CCNY) in 1942. While at CCNY, Murray focused on courses in mathematical physics and thermodynamics. He was inspired by one of his analysis professors, Emil Post, who encouraged him to continue studying mathematics. After completing a bachelor of science degree at CCNY in 1946, he entered the graduate program in mathematics at Cornell University in 1946. Although having no previous experience in probability and statistics, he was exposed to two giants in the field — William Feller and Marc Kac — who in turn generated a great deal of synergy in probability that rubbed off on Murray. They were exciting times at Cornell, which attracted a number of promising young visitors, including Joseph Doob, Monroe Donsker, Donald Darling, and Kai Lai Chung. Murray’s first courses in probability theory and mathematical statistics were taught by Feller. In the end, he chose Kac as his Ph.D. supervisor because he found his personality more compatible with his own and because he allowed students more independence in their choice of research topics.
During his undergraduate years, Murray had a chance encounter with what was to be his future wife, Adylin Lipson, at the Fordham Road Library in the Bronx. Apparently, Adylin, known as Ady, did not make a positive first impression on Murray’s mother. She blamed Ady for enticing Murray into walking home from the library in the rain, whereupon he developed a severe cold. Ady was also born and raised in the Bronx, received a bachelor’s degree in social work from Hunter College in 1947 and would later earn a master’s degree from San Diego State University in 1971. They were married in Ithaca in 1949, shortly after Murray received his Ph.D., and the couple spent one year in Ithaca before Murray began his academic career at the University of Chicago.
Career
After entering graduate school at Cornell, Murray’s interest in analysis and thermodynamics naturally attracted him to Kac’s work on statistical mechanics. He chose to work with Kac because he “sort of left you alone without saying you’ve got to do this or that so forth and so on. He let you go your own way.” This advising philosophy would serve as Murray’s mantra in mentoring his own Ph.D. students. As Murray described it, his dissertation, “On distributions of certain Wiener functionals” was “an attempt to mildly generalize some results of Kac” that were related to the seminal Feynman–Kac formula. The thesis, published in the Transactions of the American Mathematics Society under the title “On a class of Markov processes,” derived properties of the Laplace transform of the distribution of certain integral functionals of Brownian motion. The resulting transform is found as a solution to a type of partial differential equation. Following his defense, Murray remained at Cornell for another year, supported by Kac’s Office of Naval Research grant.
After finishing his work at Cornell, academic positions were scarce, and Murray was about to accept a government job when the University of Chicago stepped up and offered him a position in their statistics group. Murray believed that Kac likely played a behind-the-scenes role in making this connection. Murray joined the Committee of Statistics, the precursor to the Department of Statistics, at Chicago, that was then headed by W. Allen Wallis and included a number of budding young researchers that included Jimmie Savage, Leo Goodman, Raghu Raj Bahadur, William Kruskal, and Charles Stein. It was at Chicago in 1951–52, when Murray first met Ulf Grenander, a young Swedish scholar. They would strike up a short but productive collaboration that produced a number of highly influential time series papers dealing primarily with estimation in the spectral domain. One of their few time domain papers considered estimation of a linear regression model with time series errors. They provided conditions on the regressors and the spectrum of the error process in order for ordinary least squares (OLS) estimates to be asymptotically efficient. This is one of the fundamental results in times series analysis. Much of their collaborative work appeared in their much-acclaimed 1957 book Statistical Analysis of Time Series. This book became a must-read for both practitioners and researchers in time series for decades. With the large number of bright young researchers and an active visitors program, the environment at Chicago was nearly ideal for someone like Murray. In addition to writing a couple of papers with visiting scholar Joe Hodges, he was also inspired by Bahadur. Murray credits his discussions with Bahadur at the beginning of his “little paper” on nonparametric density estimation [3], which appeared in the Annals of Mathematical Statistics in 1956. Of course this “little paper,” which currently has around 5,000 citations, was the first to introduce kernel density estimation. This has now become one of the standard nonparametric estimation techniques and generated a rich and important line of research activity.
In 1956, Murray left Chicago for the mathematics department at Indiana University, where he struck up a collaboration with Julius Blum. The stint at Indiana lasted only a few years before he landed at Brown University in 1959. Returning to the East Coast had many attractions, including the opportunity to meet regularly with researchers at nearby Bell Laboratories. There he interacted with David Slepian and others who triggered his interest in modeling physical science phenomena. Motivated by a problem in human vision, Murray and Slepian wrote a paper on a nth order Markov process in which \( n \) variables are independent but \( (n+1) \) variables are dependent [5]. While visiting Bell Labs, Murray met one of John Tukey’s Ph.D. students, David Brillinger, who was working on higher-order properties of times series models. This interaction developed into a series of collaborative papers on higher-order spectra.
During his Brown years, Murray had an opportunity to visit the Statistics Department at Columbia University. It was there that he wrote perhaps his most well-known paper, “A central limit theorem and a strong mixing condition” [2], which introduced the strong mixing condition. This work was motivated in part by an idea of Serge Bernstein that broke up sequences of random variables into nearly independent blocks of random variables. The condition on the blocks, which specifies that they become roughly independent as the blocks grow in size and the separation increases, is in essence the strong mixing condition.
Murray left Brown for the newly created University of California at San Diego (UCSD) in 1964. Scripps Institution of Oceanography predated the university, and its strong research group that was analyzing a multitude of time series, not to mention the San Diego weather, was a major attraction. Murray arrived in 1964, one year before the first undergraduates were admitted. These were heady times to be part of the new faculty that in a short time would turn UCSD into a world-class research university. UCSD was fortunate to have attracted a number of talented young probabilists and continues to this day to have a major presence in probability theory.
Technical contributions
Strong mixing and the central limit theorem
Kernel density estimation
Rosenblatt’s 1956 seminal paper on nonparametric density estimation
introduces the kernel density estimate
[3].
The objective of this
paper was to construct an estimate of the probability density function
(pdf) \( f(x) \) from a sample of independent and identically distributed
random variables \( X_1,\dots, X_n \) having density \( f \). The motivation
for the kernel estimate comes from taking the difference of the
empirical distribution function
\[
F_n (x) = \frac{\# \{i\leq n:X_i \leq x\}}{n}.
\]
So an obvious estimate of the pdf is given by the difference
\[
f_n(y) = \frac{F_n(y+h) - F_n (y-h)}{2h},
\]
where \( h=h_n \) is the bandwidth that is a function of the sample size \( n \) that converges to 0
with increasing \( n \). Using well-known properties of \( F_n \), it is straightforward to derive the
corresponding statistical properties of \( f_n \). Assuming the existence of three derivatives of \( f \),
he showed that the optimal choice of the bandwidth that minimizes the asymptotic integrated mean squared error,
\[
\int^{\infty}_{-\infty} E|f_n(y)-f(y)|^2 \, dy,
\]
is
\[
h_n=kn^{-\tfrac{1}{5}}, \quad\text{where}\quad k =
\biggl[\frac{9}{2\int^{\infty}_{-\infty}|f^{\prime\prime}(y)|^2\,
dy}\biggr]^{\tfrac{1}{5}}.
\]
This naive estimate is an example of a kernel density estimate in which the kernel
\( w(x)=\tfrac{1}{2} \), for \( |x|\leq 1 \), and 0, otherwise. More generally, this paper considered kernel
density estimates given by
\[
f_n(y)=\int^{\infty}_{-\infty}w_n(y-u)\,dF_n(u)
=\frac{1}{n}\sum^{n}_{t=1}w_n(y-X_t),
\]
where \( w_n(u)=\frac{1}{h}w\bigl(\frac{u}{h}\bigr) \), and \( w\geq 0 \)
satisfies
\[
\int^{\infty}_{-\infty}w(u)\,du = 1,\quad
\int^{\infty}_{-\infty} uw(u)\,du = 0, \quad \text{and}\quad
\int^{\infty}_{-\infty} |u|^3 w(u)\,du < \infty.
\]
The mean-squared error of these estimates is of order
\( 0 (n^{-4/5}) \).
This basic idea was extended by many others to
additional sorts of problems, including nonparametric regression
estimates. A great deal of effort went into designing optimal
kernel functions. Rosenblatt continued his research on nonparametric density estimation
throughout much of his research life, and one of his most noted papers
is with
Bickel
on
global measures of density estimates, which appeared in the
Annals of Statistics in 1973
[6].
The idea was to consider the kernel density estimate (a) for constructing uniform confidence bands for \( f \) of the form
\( f_n \pm c_n \sqrt{f_n} \)
and (b) for constructing goodness of fit tests for
the null hypothesis \( H:f=f_0 \) using the test statistic
\[
\max_x\frac{| f_n(x) - f_0(x)|}{\sqrt{f_0(x)}}.
\]
The limit theory was based on the heuristic that the process
\[
U_n(x):=\sqrt{nh_n}\,\frac{ f_n(x)-f(x)}{\sqrt{f(x)}},
\]
which
was asymptotically independent for distinct and fixed values of \( x \), behaved locally like
a stationary Gaussian process. The resulting test statistic could then be described as a
maxima of a Gaussian process over an increasing interval on the real line. The results
were ahead of their time, as the Bickel and Rosenblatt paper also made detailed use of
the limiting distribution (Gumbel) of the maxima of a Gaussian process under different
smoothness conditions.
Limit theory for long memory processes
In his 1960 Berkeley Symposium paper, “Independence and Dependence,” Rosenblatt considered limit theory for partial sums of stationary sequences exhibiting long memory. In such a setting, the CLT he had established for strongly mixing sequences no longer applied. In this paper, he considers a function of a stationary Gaussian process \( \{Z_t\} \) with mean 0 and autocovariance function \[ r(h) = (1+h^2)^{-\tfrac{\gamma}{2}} \] with \( 0 < \gamma < \frac12 \). He chose the simplest nonlinear function of \( \{Z_t\} \) (linear functions would again be Gaussian and not of interest) given by \( X_t=Z^2_t-1 \), which again is a mean zero stationary time series. But this process is not strong mixing. The spectral density function of the \( Z_t \) has a singularlity of the form \( |\omega|^{\gamma-1} \) in a neighborhood of the origin. Rosenblatt then established the limit distribution of the normalized partial sums \( n^{\gamma-1}\sum^n_{t=1}X_t \). Given the long-memory of the process, the variance of the partial sums grows at a much slower rate and hence the normalization is much smaller, \( n^{\gamma-1} \) compared to the usual \( n^{1/2} \). In addition the limit distribution is nonnormal. This example has generated a great deal of interest, and it was picked up by others, specifically M. S. Taqqu [e2] and R. L. Dobrushin and P. Major [e3]. They extended this notion to a wide range of processes and were able to classify functional limits of the partial sum process.
Bispectrum
Much of the early work in time series had a strong frequency domain flavor, with focus on the second-order spectrum. It was found that second-order models were not sufficiently rich to model a range of physical phenomena. In the late 1950s and early 1960s, A. Kolmogorov urged the young scholars V. P. Leonov and A. N. Shiryaev to consider higher-order models with a view towards describing nonlinear behavior. Such models require analysis that goes beyond the standard spectral analysis, which only dealt with second-order properties (covariance function) of the process. In the mid-1960s, Rosenblatt began to work in a similar vein on higher-order spectra. Around this time, a promising Princeton Ph.D. student, David Brillinger, who was working at Bell Labs, was inspired by Rosenblatt’s work and an important collaboration was formed. In a series of influential papers in 1967, Brillinger and Rosenblatt developed the asymptotic theory for estimates of the cumulant spectrum under mixing conditions. This research set the stage for identification of nonlinear time series models. Rosenblatt continued working on higher-order spectral theory throughout his professional career. These ideas played a key role in modeling nonminimum phase and nonGaussian linear processes. These ideas also played a role in his books Stationary Sequences and Random Fields and Gaussian and Non-Gaussian Linear Time Series and Random Fields.
Other major contributions
Rosenblatt made major contributions in a number of other areas as well. Much of this work seemed to be motivated by a conjecture by N. Wiener [e1] that gets at the heart of general time series modeling. The setup is the following. If \( \{X_t\} \) is a stationary process with \( \mathcal{B}_n = \sigma(X_j, j\leq n) \) then when does the process have a one-sided causal representation, i.e., when does \( X_n \) have the representation, \[ X_n = f (\xi_n,\xi_{n-1},\dots), \] for some iid sequence \( \{\xi_t\} \). Wiener conjectured that a necessary and sufficient condition for such a representation is that the backward tail \( \sigma \)-field be trivial, \( \mathcal{B}_{-\infty} = \bigcap_n\mathcal{B}_n=\{\psi,\Omega\} \). Rosenblatt [4] showed this was true for Markov chains with a countable state space. Rosenblatt [8], [7] discussed when this conjecture holds and fails. Aside from the full scope of when Wiener’s conjecture holds, one can see traces of this notion in many of Rosenblatt’s research areas. This includes his interest on limits of convolutions of probability measures on compact semigroups and in the deconvolution problem. The basic setting of the deconvolution problem is that the observations \( X_t \) come from a linear system driven by iid noise, i.e., \[ X_t = \sum^{\infty}_{j=-\infty}\psi_j\xi_{t-j}, \] where the \( \{\psi_j\} \) represents a linear filter and \( \{\xi_t\} \) is iid noise. Then the question is, when can one recover the noise sequence? If the filter is known, this is relatively straightforward. But if the filter is unknown, then the goal is to deconvolve the filter from the noise. In a case in which the noise is Gaussian, then there is an identification problem. Rosenblatt studied this problem in the nonGaussian setting using higher-order spectra and likelihood methods when the distribution of the noise is known. It is of interest to know when the filter is minimum phase, which corresponds to the case that the representation is one sided, \( X_t=\sum^{\infty}_{j=0}\psi_j\xi_{t-j} \), depending only on the past of \( \xi_s \), \( s\leq t \). So Wiener’s conjecture comes full circle to modern time-series modeling.
Finally, there is the three-page Rosenblatt paper, “Remarks on a multivariate transformation” in the Annals of Mathematical Statistics [1]. The rather straightforward transformation described in this paper maps a random vector \( X=(X_1,\dots,X_k) \) with an absolutely continuous distribution into a random vector in which the components are iid uniform on \( (0,1) \). This is the multivariate analogue of the standard one-dimensional probability integral transform. Although this is not a deep result, it has proven extremely handy in modern statistics, especially in the context of copula modeling and goodness of fit of multivariate distributions. This paper has nearly 3,000 citations.
After Murray became Distinguished Professor Emeritus at UCSD in 1994, he remained actively engaged in research until the last few years of his life. After a courageous fight with cancer, Ady passed away in 2009. The Rosenblatts had two children, daughter Karin (currently of Champaign, Illinois) and son Daniel (of Live Oak, Texas). Murray advised twenty-two dissertations, and to many of these Ph.D. students, he and Ady served as surrogate parents during their graduate school years. He was there for his students with great encouragement and support. In celebration of Murray’s 90th birthday, the Murray and Adylin Rosenblatt Endowed Lecture Series in Applied Mathematics was created at UCSD in 2016.1
Concluding remarks
Murray Rosenblatt had amazing intuition and insight about random phenomena — it was a sixth sense about how things should work. He was a scholar who seemed to never have forgotten a historical fact or theorem. To his Ph.D. students, he appeared to be the most knowledgeable person, on topics from geography to history to the physical sciences and mathematics, that they ever encountered. While Murray had a soothing and relaxing style of lecturing, he could be laconic in both his writings and verbal communication. The latter could be especially perplexing to new researchers trying to decode his thoughts and insights on various mathematics problems. It was not uncommon for a former Ph.D. student or young colleague to only fully grasp what Rosenblatt had clearly understood and tried to convey years earlier: “Oh, that is what Murray meant” was a common thought. Murray’s advising approach replicated the one he experienced with Kac. He would let his students work on what they wanted and left them to their own devices. But he offered clear guidance, especially when students were struggling to find their way. He made all of his students feel important regardless of their aptitude and provided behind the scenes support in job searches and other endeavors to help further their careers.
Later in life Rosenblatt became fascinated with Chinese silk and became a quasi-expert, an expression he liked to use, on all things related to the manufacturing and production of the fabric. He loved going on long walks and hiking in the mountains whenever he had the chance. Even at an advanced age, it was difficult for his younger companions to keep up with his pace. In later years, Murray and Ady very much enjoyed spending holidays in Hawaii with his brother David and sister-in-law Joan.
Stigler’s law of eponymy states that no scientific discovery is named after its original discoverer. Murray would occasionally echo sentiments similar to this law to his Ph.D. students, who although they were enthusiastic, they were naive and did not know better [e4]. So it is somewhat ironic that a number of terms in probability and statistics now bear the Rosenblatt name. This includes the Rosenblatt transformation and the Rosenblatt process, and sometimes strong mixing is referred to as Rosenblatt mixing. Murray also tended to eschew labels. Was he a probabilist, a statistician, a mathematician? He spent his entire academic life in a department of mathematics as opposed to a statistics or engineering department. His affinity towards mathematics was never more evident than during one of our last conversations when I asked, “What are you thinking about these days?” Murray responded, “I am thinking about the foundations of mathematics.” He then pointed me to the book on his desk, Princeton Companion to Mathematics, a heavy read that he was currently working through.
Question answered — always a mathematician.
Major honors
1955 | Fellow, Institute of Mathematical Statistics |
1965–66 | Guggenheim Fellow |
1970 | Wald Lectures |
1971–72 | Guggenheim Fellow |
1975 | Fellow of the American Association for the Advancement of Science |
1979 | Overseas Fellow, Churchill College, Cambridge University |
1984 | Elected to the National Academy of Sciences |
2009 | Fellow of the Society for Industrial and Applied Mathematics |
2014 | Fellow of the American Mathematical Society |